library(tidyverse)
library(patchwork)
source("R/helpers.R")
source("R/forest_model.R")
set.seed(100)
19 Applying Bayesian calibration methods to a process model
In Chapter 14, you learned how to estimate parameter values using Bayesian methods. Now, we will apply these methods to estimate parameters in our forest process model from Chapter 16. Recall that the advantage of the Bayesian approach is that it estimates the parameters given the data, whereas likelihood approaches technically estimate the data given the parameters.
19.1 Running MCMC on the forest process model
<- "OSBS" site
Read in observations
<- read_csv("data/site_carbon_data.csv", show_col_types = FALSE) obs
Set up dates of simulation, parameters, initial conditions, and meteorology inputs
<- seq(as_date("2022-01-01"), Sys.Date() - 2, by = "1 day")
sim_dates
<- 1
ens_members <- list()
params $alpha <- rep(0.02, ens_members)
params$SLA <- rep(4.74, ens_members)
params$leaf_frac <- rep(0.315, ens_members)
params$Ra_frac <- rep(0.5, ens_members)
params$Rbasal <- rep(0.002, ens_members)
params$Q10 <- rep(2.1, ens_members)
params$litterfall_rate <- rep(1/(2.0*365), ens_members) #Two year leaf lifespan
params$litterfall_start <- rep(250, ens_members)
params$litterfall_length<- rep(60, ens_members)
params$mortality <- rep(0.00015, ens_members) #Wood lives about 18 years on average (all trees, branches, roots, course roots)
params$sigma.leaf <- rep(0.0, ens_members) #0.01
params$sigma.wood <- rep(0.0, ens_members) #0.01 ## wood biomass
params$sigma.soil <- rep(0.0, ens_members)# 0.01
params<- as.data.frame(params)
params
<- rep(NA, 3)
state_init
1] <- obs |>
state_init[filter(datetime %in% sim_dates,
== "lai") |>
variable na.omit() |>
slice(1) |>
mutate(observation = observation / (mean(params$SLA) * 0.1)) |>
pull(observation)
2] <- obs |>
state_init[filter(variable == "wood",
%in% sim_dates) |>
datetime na.omit() |>
slice(1) |>
pull(observation)
3] <- obs |>
state_init[filter(variable == "som") |>
na.omit() |>
slice(1) |>
pull(observation)
<- get_historical_met(site = site, sim_dates, use_mean = TRUE)
inputs <- assign_met_ensembles(inputs, ens_members) inputs_ensemble
Set up MCMC configuration
#Set MCMC Configuration
<- 1500
num_iter <- -10000000000
log_likelihood_prior_current <- 0
accept
#Initialize chain
<- 3
num_pars <- c(0.001, 0.0002, 1)
jump_params <- array(NA, dim = c(num_pars, num_iter))
fit_params 1, 1] <- params$alpha
fit_params[2, 1] <- params$Rbasal
fit_params[3, 1] <- params$litterfall_start
fit_params[<- c(0.029, 0.002, 200)
prior_mean <- c(0.005, 0.0005, 10) prior_sd
Run MCMC
for(iter in 2:num_iter){
#Loop through parameter value
for(j in 1:num_pars){
<- fit_params[, iter - 1]
proposed_pars <- rnorm(1, mean = fit_params[j, iter - 1], sd = jump_params[j])
proposed_pars[j]
<- dnorm(proposed_pars[1], mean = prior_mean[1], sd = prior_sd[1], log = TRUE) +
log_prior dnorm(proposed_pars[2], mean = prior_mean[2], sd = prior_sd[2], log = TRUE) +
dnorm(proposed_pars[3], mean = prior_mean[3], sd = prior_sd[3], log = TRUE)
$alpha <- proposed_pars[1]
params$Rbasal <- proposed_pars[2]
params$litterfall_start <- proposed_pars[3]
params
#Set initial conditions
<- array(NA, dim = c(length(sim_dates), ens_members, 12)) #12 is the number of outputs
output 1, , 1] <- state_init[1]
output[1, , 2] <- state_init[2]
output[1, , 3] <- state_init[3]
output[
for(t in 2:length(sim_dates)){
<- forest_model(t,
output[t, , ] states = matrix(output[t-1 , , 1:3], nrow = ens_members) ,
parms = params,
inputs = matrix(inputs_ensemble[t ,, ], nrow = ens_members))
}
<- output_to_df(output, sim_dates, sim_name = "fitting")
output_df
<- combine_model_obs(output_df,
combined_output_obs
obs,variables = c("lai", "wood", "som", "nee"),
sds = c(0.1, 1, 20, 0.01))
<- sum(dnorm(x = combined_output_obs$observation,
log_likelihood mean = combined_output_obs$prediction,
sd = combined_output_obs$sds, log = TRUE))
<- log_prior + log_likelihood
log_likelihood_prior_proposed
<- exp(log_likelihood_prior_proposed - log_likelihood_prior_current)
z
<- runif(1, min = 0, max = 1)
r
if(z > r){
<- proposed_pars[j]
fit_params[j, iter] <- log_likelihood_prior_proposed
log_likelihood_prior_current <- accept + 1
accept else{
}<- fit_params[j, iter - 1]
fit_params[j, iter] <- log_likelihood_prior_current #this calculation isn't necessary but is here to show you the logic
log_likelihood_prior_current
}
} }
Examine acceptance rate (goal is 23-45%)
/ (num_iter * num_pars) accept
[1] 0.3506667
Process MCMC chain by removing the first 500 iterations and pivoting to a long format
<- 500
nburn <- tibble(iter = nburn:num_iter,
parameter_MCMC alpha = fit_params[1, nburn:num_iter],
Rbasal = fit_params[2, nburn:num_iter],
litterfall_start = fit_params[3, nburn:num_iter]) %>%
pivot_longer(-iter, values_to = "value", names_to = "parameter")
<- ggplot(parameter_MCMC, aes(x = iter, y = value)) +
p1 geom_line() +
facet_wrap(~parameter, scales = "free") +
theme_bw()
<- ggplot(parameter_MCMC, aes(x = value)) +
p2 geom_histogram() +
facet_wrap(~parameter, scales = "free") +
theme_bw()
/ p2 p1
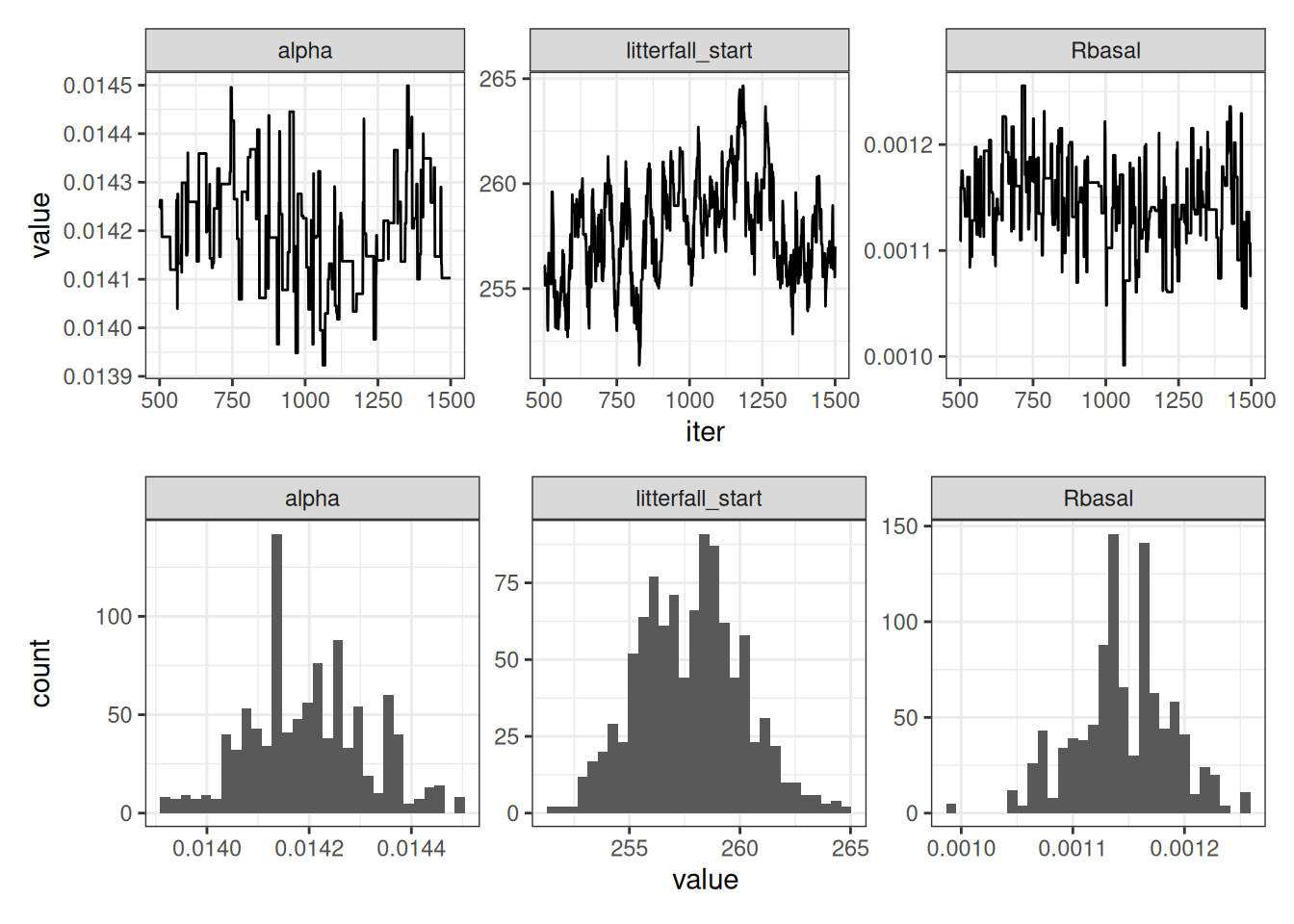
19.2 Examining the influence of parameter optimization on model predictions
19.2.1 Simulation with prior parameter distributions
<- 100
ens_members
<- assign_met_ensembles(inputs, ens_members)
inputs_ensemble
#Set initial conditions
<- array(NA, dim = c(length(sim_dates), ens_members, 12)) #12 is the number of outputs
output 1, , 1] <- state_init[1]
output[1, , 2] <- state_init[2]
output[1, , 3] <- state_init[3]
output[
<- list()
params $alpha <- rep(0.02, ens_members)
params$SLA <- rep(4.74, ens_members)
params$leaf_frac <- rep(0.315, ens_members)
params$Ra_frac <- rep(0.5, ens_members)
params$Rbasal <- rep(0.002, ens_members)
params$Q10 <- rep(2.1, ens_members)
params$litterfall_rate <- rep(1/(2.0*365), ens_members) #Two year leaf lifespan
params$litterfall_start <- rep(200, ens_members)
params$litterfall_length<- rep(70, ens_members)
params$mortality <- rep(0.00015, ens_members) #Wood lives about 18 years on average (all trees, branches, roots, course roots)
params$sigma.leaf <- rep(0.0, ens_members) #0.01
params$sigma.wood <- rep(0.0, ens_members) #0.01 ## wood biomass
params$sigma.soil <- rep(0.0, ens_members)# 0.01
params<- as.data.frame(params)
params
#Replace parameters with prior distribution
$alpha <- rnorm(ens_members, mean = prior_mean[1], sd = prior_sd[1])
params$Rbasal <- rnorm(ens_members, mean = prior_mean[2], sd = prior_sd[2])
params$litterfall_start <- rnorm(ens_members, mean = prior_mean[3], sd = prior_sd[3])
params
for(t in 2:length(sim_dates)){
<- forest_model(t,
output[t, , ] states = matrix(output[t-1 , , 1:3], nrow = ens_members) ,
parms = params,
inputs = matrix(inputs_ensemble[t ,, ], nrow = ens_members))
}
<- output_to_df(output, sim_dates, sim_name = "using prior") output_df_no_optim
19.2.2 Simulation with posterior parameter distributions
#Set initial conditions
<- array(NA, dim = c(length(sim_dates), ens_members, 12)) #12 is the number of outputs
output 1, , 1] <- state_init[1]
output[1, , 2] <- state_init[2]
output[1, , 3] <- state_init[3]
output[
# Sample from posterior distributions. Use the same index for each
<- sample(nburn:num_iter, ens_members, replace = TRUE)
index
$alpha <- fit_params[1, index]
params$Rbasal <- fit_params[2, index]
params$litterfall_start <- fit_params[3, index]
params
for(t in 2:length(sim_dates)){
<- forest_model(t,
output[t, , ] states = matrix(output[t-1 , , 1:3], nrow = ens_members) ,
parms = params,
inputs = matrix(inputs_ensemble[t ,, ], nrow = ens_members))
}
<- output_to_df(output, sim_dates, sim_name = "using posteriors") output_df_optim
19.2.3 Visualize the influence of optimization
Figure 19.2 shows a simulation that uses the prior distribution and a simulation that uses the posterior distribution. This highlights the constraint provided by the data on the model predictions.
<- obs |>
obs_filtered filter(datetime > min(output_df_no_optim$datetime))
bind_rows(output_df_no_optim, output_df_optim) |>
summarise(median = median(prediction, na.rm = TRUE),
upper90 = quantile(prediction, 0.95, na.rm = TRUE),
lower90 = quantile(prediction, 0.05, na.rm = TRUE),
.by = c("datetime", "variable", "model_id")) |>
filter(variable %in% c("lai", "wood", "som", "nee")) |>
ggplot(aes(x = datetime)) +
geom_ribbon(aes(ymin = lower90, ymax = upper90, fill = model_id), alpha = 0.7) +
geom_line(aes(y = median, color = model_id)) +
geom_point(data = obs_filtered, aes(x = datetime, y = observation), color = "blue", alpha = 0.5) +
facet_wrap(~variable, scale = "free", ncol = 1) +
theme_bw()
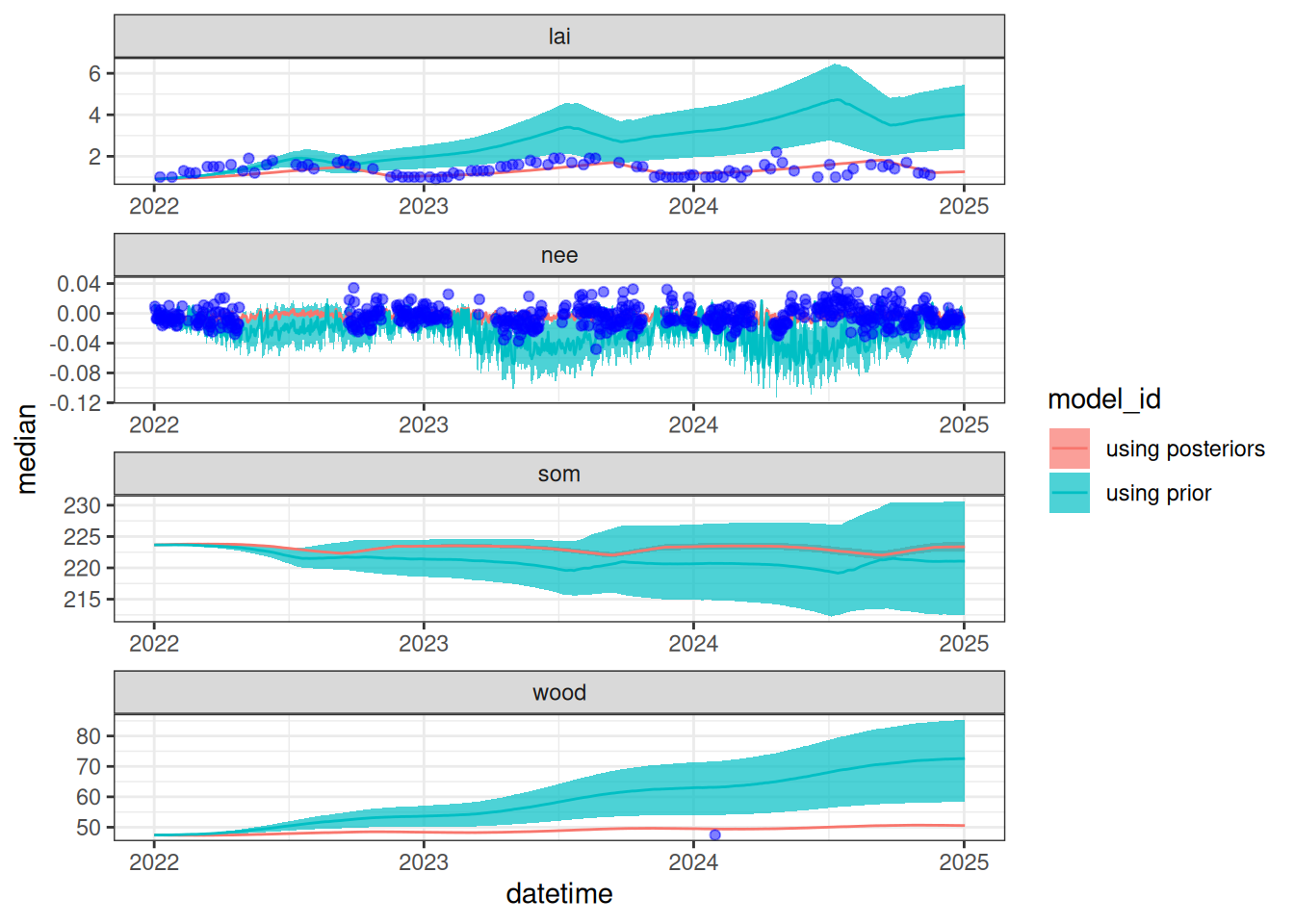
19.2.4 Save posteriors for future use
The calibrated parameter chains will be used in later chapters.
write_csv(parameter_MCMC, "data/saved_parameter_chain.csv")